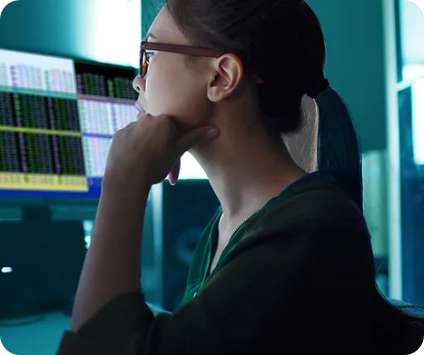
- October 23, 2024
- 1. Introduction
- 2. Significance and Scope
- 3. Types of Data Analytics
- 4. Importance of Data Analytics
- 5. Top 7 Applications of Data Analytics in 2024
- 6. Benefits of Data Analytics
- 7. Data Analytics Trends
- 8. FAQs on Data Analytics:
- 9. Leveraging Data Analytics for Retail Excellence
- 10. The Evolving Landscape of Data Analytics in Retail
- 11. FAQs on Retail Data Analytics:
1. Introduction
Data analytics is the process of examining and interpreting data to derive meaningful insights, make informed decisions, and uncover valuable patterns. This section serves as a gateway for both beginners and enthusiasts, offering a comprehensive overview of the field. We explore the significance of data analytics in today’s data-driven world, outlining its applications across various industries. From business intelligence to predictive modelling, the blog navigates through key concepts, tools, and methodologies, providing readers with a solid understanding of the landscape. Whether you’re a novice seeking to grasp the basics or a professional aiming to enhance your skills, this introduction sets the stage for a deeper exploration into the dynamic realm of data analytics.
2. Significance and Scope
In the realm of data analytics, significance and scope are pivotal aspects that shape its impact and application. Understanding the significance delves into why data analytics is a trans formative force across diverse industries. It empowers businesses to glean actionable insights from vast datasets, driving informed decision-making. The scope of data analytics encompasses a wide range of applications, from optimizing operational efficiency to identifying market trends and predicting future outcomes. Its reach extends beyond business, influencing fields like healthcare, finance, and technology.
Furthermore, the scope of data analytics is continually expanding, propelled by advancements in machine learning and artificial intelligence. This evolution opens new avenues for exploration, offering solutions to previously insurmountable challenges. As organisations increasingly recognize the potential within their data, the significance and scope of data analytics become integral components in harnessing its power for innovation and strategic growth.
3. Types of Data Analytics
3.1 Descriptive Analytics
In the landscape of Types of Data Analytics, Descriptive Analytics (Heading 3) stands as a fundamental category that focuses on understanding and summarizing historical data. This analytical approach involves the examination of past data to identify patterns, trends, and key insights. Descriptive Analytics provides organizations with a retrospective view, enabling them to comprehend what has happened in their operations. Through the use of various statistical and visualization tools, such as charts and graphs, Descriptive Analytics transforms raw data into meaningful information.
This type of analytics plays a crucial role in aiding decision-makers by offering a comprehensive overview of historical performance. Businesses can utilize Descriptive Analytics to gain insights into customer behavior, operational efficiency, and overall performance metrics. By illuminating the past, organizations can make informed decisions, optimize strategies, and lay the foundation for more advanced forms of analytics, such as predictive and prescriptive analytics, thus contributing to a data-driven and informed decision-making culture.
3.2 Diagnostic Analytics
Diagnostic Analytics is instrumental in uncovering insights that go beyond surface-level observations, enabling organizations to identify the drivers behind certain trends or occurrences. By employing statistical techniques and data exploration methods, analysts can pinpoint factors that contributed to particular outcomes, facilitating a more nuanced understanding of the underlying dynamics. This type of analytics is invaluable for problem-solving and strategic decision-making, empowering businesses to address challenges proactively and optimize processes based on a thorough comprehension of causal relationships within their datasets.
3.3 Predictive Analyticsis
Predictive Analyticsis a transformative facet within the broader landscape of Types of Data Analytics. It involves the use of statistical algorithms and machine learning techniques to analyze historical data and predict future outcomes or trends. Unlike Descriptive and Diagnostic Analytics, which focus on understanding past events, Predictive Analytics is forward-looking, leveraging patterns and correlations to make informed predictions.
3.4 Prescriptive Analytics
Prescriptive Analytics is a pinnacle in the hierarchy of Types of Data Analytics, representing the most advanced and actionable form of analysis. It goes beyond the realms of Descriptive, Diagnostic, and Predictive Analytics by not only predicting future outcomes but also recommending optimal actions to achieve desired results. This sophisticated analytical approach utilizes a combination of data, mathematical models, and machine learning algorithms to provide decision-makers with actionable insights.
Prescriptive Analytics guides organizations in determining the best course of action based on the predicted future scenarios. By considering various decision options and their potential outcomes, businesses can make informed choices that align with their strategic objectives. This type of analytics is particularly valuable in complex and dynamic environments, where decision-makers need guidance on how to respond to changing conditions.
4. Importance of Data Analytics
4.1 Business Decision-Making
In the realm of data analytics, understanding historical data (as discussed in the second section) is crucial for organizations. It involves examining past data to uncover patterns and trends, facilitating informed decision-making. Similarly, delving deeper into the ‘why’ behind specific outcomes (as discussed in the third section) allows for a comprehensive understanding of
causal relationships. This type of analytics is instrumental in problem-solving and strategic decision-making.
Predictive analytics, on the other hand, takes a forward-looking approach by using statistical algorithms to forecast future trends. It enables organizations to anticipate developments, identify opportunities, and mitigate risks, fostering a proactive decision-making culture.
At the pinnacle of data analytics lies a more advanced approach—prescriptive analytics. This method not only predicts future outcomes but also recommends optimal actions to achieve desired results. It empowers organizations to make informed choices that align with their strategic objectives, especially in complex and dynamic environments.
4.2 Performance Optimization
Within the overarching theme of the importance of data analytics, performance optimization emerges as a critical facet. Data analytics empowers organizations to enhance their operational efficiency and strategic effectiveness. By systematically analyzing relevant data sets, businesses can identify inefficiencies, streamline processes, and allocate resources more effectively.
Performance optimization through data analytics extends across various aspects of an organization, from refining internal workflows to enhancing customer experiences. Through insights derived from data, organizations can pinpoint areas for improvement, implement targeted changes, and monitor the impact on performance metrics. This iterative process of analysis and optimization creates a dynamic feedback loop, fostering continuous improvement. Moreover, data analytics plays a pivotal role in adapting strategies in real time, aligning them with evolving market conditions. It enables organizations to stay agile, responsive, and competitive.
Enhancing Customer Experience
In the broader context of recognizing the importance of data analytics, enhancing customer experience stands out as a pivotal aspect. Data analytics plays a transformative role in understanding customer behaviors, preferences, and expectations. By leveraging data-driven insights, organizations can tailor their products, services, and interactions to meet and exceed customer expectations.
Enhancing customer experience through data analytics involves analyzing customer data to identify patterns, predict future needs, and personalize interactions. This personalized approach not only fosters customer satisfaction but also contributes to customer loyalty and advocacy. Businesses can use analytics to create targeted marketing campaigns, optimize the user interface of digital platforms, and provide personalized recommendations.
5. Top 7 Applications of Data Analytics in 2024
5.1 Healthcare Industry
In the landscape of the top applications of data analytics in 2024, the healthcare industry takes center stage as a transformative and crucial domain. Data analytics plays a pivotal role in revolutionizing healthcare by optimizing processes, improving patient outcomes, and enhancing overall efficiency.
One primary application lies in predictive analytics for patient care. By analyzing vast datasets, healthcare providers can predict disease trends, identify high-risk patients, and personalize treatment plans. This proactive approach not only improves patient outcomes but also aids in resource allocation and healthcare planning.
Additionally, data analytics is instrumental in streamlining operational workflows, reducing costs, and improving the allocation of resources within healthcare organizations. From optimizing supply chain management to enhancing patient scheduling systems, analytics contributes to a more efficient and cost-effective healthcare ecosystem.
5.2 Financial Services
In the unfolding landscape of the top applications of data analytics in 2024, the financial services sector is a prominent arena where analytics reshapes operations and strategies. Data analytics in the financial industry is instrumental in fraud detection, risk management, and customer-centric services.
One key application is in fraud detection and prevention. Advanced analytics algorithms analyze transaction patterns and detect real-time anomalies, providing a robust defense against fraudulent activities. This not only safeguards financial institutions but also enhances trust among customers.
Risk management is another critical facet where data analytics plays a pivotal role. By leveraging predictive analytics, financial institutions can assess and mitigate risks more effectively, whether in lending, investment decisions, or market fluctuations. This proactive approach enhances decision-making and overall stability within the financial sector.
5.3 Marketing and Advertising
In the dynamic landscape of the top applications of data analytics in 2024, the domain of Marketing and Advertising takes a prominent position, showcasing the transformative power of analytics in optimizing strategies and enhancing customer engagement.
Data analytics in marketing and advertising is pivotal for targeted and personalized campaigns. By analyzing vast datasets encompassing consumer behavior, preferences, and demographics, businesses can tailor their marketing efforts with precision, delivering messages that resonate with specific audiences. This not only maximizes the impact of marketing initiatives but also improves the return on investment.
Predictive analytics plays a crucial role in forecasting trends and anticipating consumer needs. This enables marketers to stay ahead of the curve, adjusting strategies in real-time to capitalize on emerging opportunities.
The integration of analytics in marketing also extends to customer journey analysis, enabling businesses to understand the complete lifecycle of customer interactions. This insight facilitates the optimization of touchpoints, refining the overall customer experience and fostering brand loyalty.
5.4 Supply Chain and Logistics
Within the realm of the top applications of data analytics in 2024, Supply Chain and Logistics emerged as a pivotal domain where analytics is driving transformative advancements. Data analytics is revolutionizing how businesses manage and optimize their supply chains, leading to improved efficiency, cost savings, and enhanced responsiveness.
One key application lies in demand forecasting. By analyzing historical data and market trends, businesses can predict future demand more accurately, allowing for proactive inventory management and reducing the risk of stockouts or excess inventory. This, in turn, streamlines the entire supply chain process.
Route optimization is another critical aspect where data analytics plays a central role. Algorithms analyze various factors, including traffic patterns and delivery schedules, to optimize the most efficient routes for transportation. This not only reduces operational costs but also minimizes environmental impact.
5.5 Cybersecurity
In the landscape of the top applications of data analytics in 2024, the realm of cybersecurity emerges as a critical domain where analytics plays a pivotal role in safeguarding digital assets and mitigating evolving cyber threats.
Data analytics in cybersecurity involves the systematic analysis of vast datasets to identify patterns, anomalies, and potential security breaches. By leveraging advanced analytics algorithms, organizations can detect unusual activities in real-time, enabling proactive responses to potential cyber threats.
Predictive analytics is crucial in cybersecurity for forecasting potential vulnerabilities and emerging attack vectors. This proactive approach allows security professionals to fortify defenses and stay one step ahead of cyber adversaries.
5.6 Telecommunications
Data analytics in telecommunications is paramount for optimizing network performance. Through the analysis of extensive datasets related to network usage, traffic patterns, and performance metrics, telecom companies can fine-tune their infrastructure. This optimization ensures seamless connectivity, reduces downtime, and enhances overall network efficiency, contributing to a more reliable and robust telecommunications ecosystem.
Customer experience takes center stage in the application of data analytics within the telecommunications industry. By delving into customer data, including usage patterns and preferences, providers can tailor services and offer personalized plans. This not only fosters customer satisfaction but also contributes to customer loyalty in a highly competitive market.
5.7 Social Media and Entertainment
Data analytics in Social Media and Entertainment is instrumental in content personalization. By analyzing user preferences, engagement patterns, and demographic information, platforms can curate personalized content feeds. This enhances user satisfaction, engagement, and the overall user experience.
Predictive analytics is crucial for anticipating content trends and user behaviors. Platforms leverage predictive models to forecast what content users are likely to engage with, enabling them to stay ahead of trends and optimize content strategies in real time.
6. Benefits of Data Analytics
6.1 Improved Decision-Making
In the context of the overarching theme highlighting the benefits of data analytics, one standout advantage is the significant improvement it brings to decision-making processes. Data analytics empowers organizations by providing insights derived from comprehensive data analysis, leading to more informed and strategic decision-making.
By leveraging historical data, trends, and patterns, decision-makers gain a deeper understanding of past performance and can anticipate future outcomes. Descriptive analytics aids in summarizing historical data, while diagnostic analytics delves into the ‘why’ behind specific events, offering a nuanced understanding of causal relationships.
Moreover, predictive analytics enables organizations to forecast future trends and make proactive decisions, while prescriptive analytics takes it a step further by recommending optimal actions based on predicted outcomes. This comprehensive approach equips decision-makers with a wealth of information to navigate uncertainties, identify opportunities, and address challenges effectively.
6.2 Efficient Operations
In the broader exploration of the benefits of data analytics, the realm of efficient operations stands out as a critical advantage. Data analytics plays a pivotal role in optimizing and streamlining organizational processes, leading to increased efficiency and resource utilization.
One key aspect is operational analytics, which involves the real-time analysis of data to monitor and enhance ongoing processes. By leveraging insights from operational data, organizations can identify bottlenecks, streamline workflows, and improve overall productivity.
Additionally, predictive analytics contributes to efficient operations by forecasting future trends and potential issues. This proactive approach allows organizations to anticipate demand, optimize inventory levels, and plan resource allocation effectively, preventing disruptions before they occur.
6.3 Competitive Edge
In the overarching discussion on the benefits of data analytics, a prominent advantage is the attainment of a competitive edge. Data analytics empowers organizations to gain valuable insights into market dynamics, customer behaviors, and emerging trends, positioning them strategically to outperform competitors.
Competitive intelligence, derived from data analytics, enables businesses to understand their market position, analyze competitor strategies, and identify opportunities for differentiation. By leveraging predictive analytics, organizations can foresee market trends, enabling them to proactively adjust their products, services, or strategies to stay ahead in a rapidly changing landscape.
6.4 Customer Insights
Amidst the comprehensive exploration of the benefits of data analytics, the realm of Customer Insights stands out as a transformative advantage. Data analytics provides businesses with a profound understanding of customer behaviors, preferences, and needs, facilitating the delivery of personalized and targeted experiences.
Through the lens of customer data, organizations can employ descriptive analytics to summarize historical interactions, diagnostic analytics to understand the factors influencing customer actions, and predictive analytics to anticipate future behaviors. This holistic approach enables the creation of a detailed customer profile, fostering a deeper connection and enhancing overall satisfaction.
6.5 Customer Behavior Analysis
Through the lens of Customer Behavior Analysis, retailers can leverage descriptive analytics to understand historical purchasing patterns and trends. Diagnostic analytics allows for deeper exploration, uncovering the reasons behind certain behaviors, such as the impact of promotions or seasonal changes. This comprehensive understanding is vital for retailers seeking to optimize their product offerings and marketing strategies.
Predictive analytics takes Customer Behavior Analysis a step further by forecasting future trends and preferences. Retailers can proactively adjust their inventory, marketing campaigns, and pricing strategies based on these predictions, ensuring they stay ahead in a dynamic market.
6.6 Inventory Management
Through predictive analytics, retailers can implement sophisticated inventory forecasting by analyzing historical sales data, market trends, and other relevant factors. This enables accurate predictions of demand, ensuring optimal stock levels and minimizing the risk of overstocking or understocking.
Descriptive analytics provides insights into historical inventory performance, helping retailers understand product lifecycles, identify top-selling items, and recognize patterns in consumer demand. Diagnostic analytics delves into the root causes of inventory issues, such as fluctuations in the supply chain or seasonal variations.
6.7 Personalized Marketing Strategies
In the context of data analytics in retail, Personalized Marketing Strategies emerge as a pivotal focus, utilizing insights from customer data to tailor marketing efforts and elevate the overall shopping experience.
Through Personalized Marketing Strategies, retailers leverage descriptive analytics to summarize historical customer interactions and preferences, laying the foundation for personalized experiences. Diagnostic analytics refines this understanding, uncovering patterns and factors influencing individual purchasing decisions.
Predictive analytics plays a central role in forecasting future trends and customer behaviors. By anticipating the needs and preferences of individual customers, retailers proactively tailor marketing messages, promotions, and product recommendations, enhancing customer engagement and increasing conversion rates.
6.8 Sales Forecasting
Sales Forecasting, facilitated by predictive analytics, involves systematically analyzing historical sales data, market trends, and various external factors to predict future sales volumes accurately. This predictive capability enables retailers to proactively align their inventory levels with expected demand, minimizing the risk of overstocking or stockouts.
Descriptive analytics plays a role in summarizing past sales performance, providing a foundational understanding of historical trends. Meanwhile, diagnostic analytics helps retailers delve deeper into the factors influencing sales fluctuations, such as seasonality or promotional activities.
7. Data Analytics Trends
7.1 AI and Machine Learning Integration
AI and ML integration in data analytics involves leveraging advanced algorithms to analyze large datasets, identify patterns, and generate predictions or recommendations. This dynamic duo enables more accurate and efficient data processing, significantly enhancing the capabilities of traditional analytics.
Through AI and ML, data analytics systems can autonomously learn and adapt to new information, continuously improving their predictive accuracy over time. This adaptive capability is precious in complex and rapidly changing business environments.
The integration of AI and ML also facilitates the automation of repetitive tasks, allowing data scientists and analysts to focus on more complex and strategic aspects of analytics. This enhances productivity and accelerates the pace at which organizations can extract actionable insights from their data.
7.2 Big Data Handling
In the evolving landscape of data analytics trends, the effective handling of Big Data emerges as a pivotal focus area, reflecting the industry’s response to the exponential growth of data volumes.
Big Data handling in data analytics involves the management and analysis of massive datasets that traditional data processing methods struggle to handle. Technologies and frameworks like Hadoop and Spark play a crucial role in enabling the storage, processing, and analysis of vast amounts of structured and unstructured data.
One key aspect of this trend is the scalability of infrastructure to accommodate growing data volumes. Cloud computing services and distributed computing architectures provide the flexibility and resources necessary to efficiently process and analyze Big Data.
7.3 Edge Analytics
Edge Analytics involves performing data analysis directly on devices or at the edge of the network where data is generated, rather than relying solely on centralized cloud-based processing. This decentralized approach brings real-time analytics closer to the data source, reducing latency and enabling quicker decision-making.
One key advantage of Edge Analytics is its ability to handle and process data locally, minimizing the need to transmit large volumes of data to centralized servers. This not only conserves bandwidth but also enhances privacy and security by keeping sensitive information closer to its origin.
This trend is particularly crucial in the era of the Internet of Things (IoT), where a multitude of devices generate massive amounts of data. Edge Analytics allows for rapid analysis of this data, providing immediate insights and responses in scenarios like industrial automation, healthcare monitoring, and smart cities.
7.4 Blockchain in Data Analytics
Amidst the unfolding trends in data analytics, the integration of Blockchain stands out as a transformative force, revolutionizing how data is stored, secured, and shared across networks.
Blockchain in data analytics involves the application of decentralized and secure ledger technology to ensure the integrity and transparency of data. By utilizing a distributed and immutable ledger, organizations can enhance data trustworthiness and reduce the risk of tampering or unauthorized alterations.
One significant application of Blockchain in data analytics is in data provenance, where the origin and history of data are securely tracked. This ensures a transparent and auditable record of data sources and modifications, fostering accountability and compliance.
8. FAQs on Data Analytics:
8.1 What are the key challenges faced in implementing Data Analytics in businesses?
Implementing Data Analytics in businesses faces challenges such as data privacy concerns, integration complexities, and the need for skilled professionals. Ensuring data security, aligning diverse data sources, and addressing the shortage of skilled talent are pivotal. Successful implementation requires strategic planning to overcome these obstacles and harness the full potential of data analytics for business growth.
8.2 How does Data Analytics impact small businesses in the retail sector?
Data Analytics significantly impacts small businesses in the retail sector by providing actionable insights for informed decision-making. It helps optimize inventory management, personalize customer experiences, and target marketing efforts effectively. Small retailers can leverage data analytics to compete with larger counterparts, enhance operational efficiency, and adapt strategies based on customer preferences, ultimately fostering growth and sustainability.
8.3 Can Data Analytics completely eliminate human errors in decision-making processes?
While Data Analytics can significantly reduce human errors in decision-making processes by providing data-driven insights, it cannot eliminate them. Humans are still involved in interpreting and acting upon the analytics results, and factors such as biased data, misinterpretation, or unexpected events may contribute to errors. Combining analytics with human judgment and validation processes is essential for more accurate decision-making.
9. Leveraging Data Analytics for Retail Excellence
9.1 Real-time Personalization in E-commerce
Real-time Personalization involves the immediate tailoring of content, product recommendations, and offers based on a user’s behavior and preferences during their current session. Through sophisticated data analytics algorithms, e-commerce platforms analyze customer interactions, purchase history, and real-time browsing patterns to dynamically adjust the content displayed.
This trend significantly enhances user engagement by presenting customers with products and promotions that align with their interests and current needs. The ability to adapt in real-time fosters a more personalized and relevant online journey, mimicking the personalized assistance one might receive in a physical store.
9.2 Blockchain in Data Analytics
In the realm of current data analytics trends, the incorporation of Blockchain technology introduces a transformative shift, providing heightened security, transparency, and trust to the data analytics domain.
Blockchain in data analytics fundamentally utilizes decentralized and distributed ledger technology to ensure the integrity and immutability of data. Each block within the chain chronicles timestamped transaction records, creating an unalterable and transparent record. This intrinsic feature substantially elevates the credibility and reliability of the data undergoing analysis.
A notable advantage lies in the secure sharing of data across multiple entities. Blockchain establishes a decentralized and tamper-resistant system, mitigating the risk of data manipulation. This aspect holds particular significance in sectors such as finance, healthcare, and supply chain, where data accuracy and security are paramount.
9.3 Omnichannel Customer Experience
In the pursuit of retail excellence through data analytics, Omnichannel Customer Experience emerges as a pivotal focus, reshaping how retailers engage with customers across diverse channels seamlessly.
Omnichannel Customer Experience, powered by data analytics, involves the holistic integration of various retail channels—online and offline—into a unified and cohesive customer journey. By leveraging customer data, retailers gain insights into individual preferences, behaviors, and purchase histories, enabling the delivery of personalized and consistent experiences across all touchpoints.
Data analytics plays a crucial role in understanding customer journeys, identifying touchpoints, and optimizing each interaction. Predictive analytics anticipates customer needs, allowing retailers to proactively tailor marketing messages, promotions, and product recommendations across multiple channels.
9.4. Predictive Inventory Management
Predictive Inventory Management, empowered by data analytics, involves the use of advanced algorithms to forecast future demand based on historical sales data, market trends, and external factors. By predicting demand patterns, retailers can optimize their inventory levels, ensuring that products are available when and where customers need them.
Data analytics enables retailers to move beyond reactive approaches to inventory management, embracing a proactive strategy that minimizes the risks of overstocking or stockouts. This predictive capability not only streamlines operational workflows but also enhances overall efficiency and cost-effectiveness.
10. The Evolving Landscape of Data Analytics in Retail
10.1 AR/VR Integration for Enhanced Shopping Experiences
In the dynamic evolution of data analytics in retail, the integration of Augmented Reality (AR) and Virtual Reality (VR) takes center stage, heralding a new era of Enhanced Shopping Experiences.
AR/VR Integration for Enhanced Shopping Experiences, fueled by data analytics, involves the amalgamation of immersive technologies into the retail landscape. Through customer data analysis, retailers gain insights into individual preferences, enabling the customization of AR/VR experiences to align with customer tastes and behaviors.
This integration transforms the traditional shopping journey by offering customers virtual try-on experiences, interactive product demonstrations, and personalized virtual storefronts. Data analytics plays a pivotal role in tailoring these experiences, ensuring they resonate with specific customer segments.
10.2 Voice Analytics for Customer Service
Voice Analytics for Customer Service, empowered by data analytics, involves the analysis of voice interactions between customers and retailers. By leveraging advanced algorithms, retailers can extract valuable insights from customer service calls, chatbots, and voice-activated devices.
Predictive analytics comes into play by identifying patterns in customer queries and feedback, enabling retailers to anticipate and proactively address potential issues. Additionally, data analytics facilitates continuous improvement by providing actionable insights for refining customer service processes based on historical data.
10.3 Sustainability and Ethical Analytics
Sustainability and Ethical Analytics, powered by data-driven insights, involve the assessment and analysis of various factors, including supply chain practices, product sourcing, and overall environmental impact. Retailers leverage data analytics to scrutinize the lifecycle of products, ensuring adherence to ethical and sustainable standards.
Data analytics provides transparency to consumers, allowing them to make informed choices aligned with their ethical values. This commitment to sustainability not only meets the increasing demand for ethical products but also fosters trust and loyalty among environmentally conscious consumers.
11. FAQs on Retail Data Analytics:
11.1 How can retail analytics ensure a seamless online-to-offline customer journey?
Retail analytics ensures a seamless online-to-offline customer journey by leveraging insights from customer data. It enables retailers to understand individual preferences, optimize inventory across channels, and offer personalized experiences. By integrating online and offline touch points, retail analytics enhances customer engagement, creating a cohesive and satisfying shopping experience across various platforms.
11.2 What role does IoT play in revolutionising data analytics for retailers?
IoT (Internet of Things) revolutionizes data analytics for retailers by providing a continuous stream of real-time data from connected devices. This data, ranging from customer preferences to inventory levels, enhances analytics accuracy. Retailers can optimize operations, personalize customer experiences, and make informed decisions based on timely insights, thereby improving efficiency and competitiveness.
11.3 How does retail data analytics accommodate privacy concerns amidst personalized marketing?
Retail data analytics addresses privacy concerns in personalized marketing by adopting robust data protection measures. Anonymizing and aggregating data protects individual identities, and compliance with privacy regulations is prioritized. Retailers implement transparent data usage policies, allowing customers to control their information. By balancing personalization with privacy safeguards, retail data analytics ensures personalized marketing without compromising individual privacy.
- 1. Introduction
- 2. Significance and Scope
- 3. Types of Data Analytics
- 4. Importance of Data Analytics
- 5. Top 7 Applications of Data Analytics in 2024
- 6. Benefits of Data Analytics
- 7. Data Analytics Trends
- 8. FAQs on Data Analytics:
- 9. Leveraging Data Analytics for Retail Excellence
- 10. The Evolving Landscape of Data Analytics in Retail
- 11. FAQs on Retail Data Analytics: