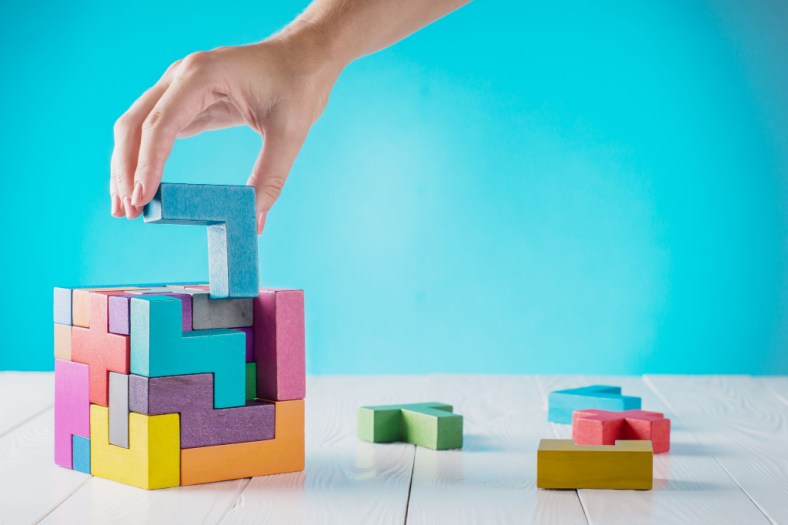
- February 21, 2025
1. High Costs and Implementation Complexity in Analytics Infrastructure
Current data-driven business operations depend on analytics infrastructure for making informed strategic decisions. Operating and managing analytics infrastructure expenses in Virginia demands considerable investment along with comprehensive technological complexity.
Gartner indicates worldwide IT spending will grow 9.8% to reach $5.61 trillion in 2025 because of data center system and software investments brought on by AI developments. Organizations face difficulties when it comes to managing their expenses effectively.
This article examines the cause of expensive and complex analytics infrastructure, important implementation challenges, and effective methods for cost reduction and process acceleration.
2. Why Analytics Infrastructure is Expensive and Complex
Building a robust analytics infrastructure requires significant investment. From hardware and software to skilled personnel, costs quickly add up. Additionally, businesses must ensure data security, compliance, and scalability, which further increases expenses.
Beyond financial factors, the complexity of setting up and managing analytics infrastructure expenses in Virginia often leads to inefficiencies. Many organizations struggle with reducing implementation complexity in data analytics, as integrating various tools and platforms requires technical expertise. Let’s examine the main factors that drive up costs and make implementation difficult.
2.1. Factors Driving High Costs in Data Analytics
The high price of analytics infrastructure stems from various cost elements. Storage solutions along with hardware systems present a financial obstacle when implementing analytic systems.
Operating businesses that collect substantial data must purchase expensive systems to manage their scalable storage needs. The expense control of analytic infrastructure is difficult when organizations neglect cost optimization in their cloud storage systems.
Second, computing power is a major expense. Running advanced analytics, machine learning models, and big data processing requires significant computing resources. Licensing fees for analytics tools also add to the costs. Proprietary software solutions often come with expensive subscriptions, making it harder for companies to manage their analytics infrastructure expenses efficiently.
Third, skilled professionals are essential for maintaining and optimizing analytics systems. Data engineers, analysts, and IT administrators command high salaries, further increasing costs. Without proper planning, businesses end up overspending on their analytics ecosystem.
2.2. The Complexity of Setting Up an Analytics Ecosystem
Creating an analytics infrastructure requires complex procedures. Businesses need to combine their information storage with processing capabilities and visualization solutions correctly to build an effective system. The problem of technical expertise stands in the way of many organizations that try to simplify their data analytics implementation process.
The process becomes more complicated due to data security and compliance requirements. As per regulation requirements including and HIPAA, organizations need to establish specific data governance policies. The implementation of such policies demands both time and financial investment.
Analytical systems must demonstrate both scalability and adaptability capabilities. Company expansion leads to expanding data requirements in their operations. Moving forward with a planned infrastructure directs organizations toward avoiding both frequent scaling challenges and increased infrastructure costs. This is why companies need effective strategies for cost optimization in analytics infrastructure while maintaining efficiency.
3. Key Challenges in Implementing Analytics Infrastructure
Beyond costs, businesses face several challenges when implementing analytics solutions. Managing data pipelines, storage, and computing power requires careful planning. Security, compliance, and integration issues further complicate the process. Without a clear strategy, organizations struggle to maintain efficiency while keeping analytics infrastructure expenses under control.
Data pipelines must handle large volumes of information efficiently. Poorly managed pipelines lead to delays, errors, and inconsistencies. Businesses also need to balance performance with cost when selecting storage and computing solutions. Without proper optimization, expenses can escalate quickly.
Security is another significant concern. Data breaches and compliance violations can result in legal and financial penalties. Companies must implement strong security measures while ensuring compliance with industry regulations. Integration challenges further add to the complexity. Connecting different analytics tools and platforms requires expertise, and any misconfiguration can create inefficiencies.
With these challenges in mind, businesses must adopt a strategic approach to manage costs and streamline implementation effectively.
3.1. Managing Storage, Compute, and Licensing Costs
Storage along with computing infrastructure and software licensing operate as the major hurdles in establishing analytics infrastructure. Modern businesses gather extensive data that necessitates both flexible and secure storage solutions due to their data volume requirements. While cloud storage offers flexibility, it can become costly without proper cost optimization in analytics infrastructure.
Computing power is another concern. Running data analytics workloads requires significant processing resources. Companies using on-premise servers face high maintenance costs, while cloud-based solutions charge based on usage, which can lead to unpredictable expenses.
Licensing costs for analytics tools also add up. Many organizations rely on proprietary software, which requires expensive subscriptions. Open-source alternatives exist but often require additional setup and maintenance efforts, increasing complexity.
3.2. Complexity in Configuring Data Pipelines
Effective data processing demands the use of data pipelines which streamline data analysis operations. The deployment process proves challenging to most organizations. Businesses must design systems that extract data from different sources through a process that includes transformation and loading stages. Correct and continuous data requires pipelines that are designed properly.
Businesses face difficulties in reducing implementation complexity in data analytics since technical skills are needed to configure pipelines. The execution of improperly built pipelines causes data discrepancies and creates operational slowdowns together with increased support expenses. Automated data pipeline management systems offer advantages however they need both specialized equipment and skilled personnel during the setup period.
4. Strategies to Reduce Costs and Simplify Implementation
To overcome these challenges, businesses need effective strategies for optimizing costs and simplifying analytics implementation. Leveraging cloud-based solutions, automating processes, and choosing cost-effective tools can significantly improve efficiency.
Organizations should assess their infrastructure and identify cost-saving opportunities. Moving workloads to the cloud can reduce on-premise hardware expenses. Implementing automation tools streamlines data workflows, reducing manual effort and errors. Businesses should also evaluate software licensing models to ensure they are not overpaying for features they do not use.
Additionally, leveraging open-source analytics tools can lower costs without sacrificing functionality. Companies must also focus on optimizing resource allocation, ensuring storage and computing resources are used efficiently. Regular monitoring and cost analysis helps prevent budget overruns and keep analytics infrastructure expenses under control.
4.1. Leveraging Cloud-Based Analytics Solutions
Cloud-based analytics solutions offer flexibility, scalability, and cost efficiency. Unlike traditional on-premise infrastructure, cloud solutions allow businesses to pay for only the resources they use. This helps in cost optimization in analytics infrastructure.
Many cloud providers offer managed analytics services that reduce the burden of maintaining infrastructure. Businesses can scale up or down based on demand, avoiding unnecessary expenses. However, it’s essential to monitor cloud usage closely to prevent unexpected charges.
Cloud security features also help businesses comply with regulations. By leveraging built-in security and compliance tools, organizations manage analytics infrastructure expenses more efficiently. Additionally, cloud-based solutions enable seamless collaboration by allowing teams to access and analyze data from anywhere. Advanced AI and machine learning integrations further enhance predictive analytics capabilities.
4.2. Automating Data Pipeline Management
Automation is key to reducing implementation complexity in data analytics. Automated data pipeline management tools streamline data processing, reducing manual effort and errors. With automation, businesses can ensure data consistency and improve efficiency.
Several tools offer low-code or no-code solutions for building and managing data pipelines. These tools reduce the need for specialized technical skills, making implementation easier. Automating data transformation and integration processes also minimizes operational costs and improves scalability. They also enhance data quality by enforcing validation rules and monitoring for anomalies. Additionally, automated pipelines enable real-time data processing, supporting faster decision-making.
5. Conclusion
Analytics infrastructure is essential for modern businesses, but high costs and complexity make implementation challenging. Without proper planning, organizations struggle with cost optimization in analytics infrastructure and reducing implementation complexity in data analytics. Managing analytics infrastructure expenses requires a strategic approach that includes cloud adoption, automation, and efficient resource allocation.
Using cloud solutions alongside automated data pipeline management can help businesses lower their expenses and achieve easier installation processes. Strategic investments in affordable data analysis tools and methods preserve lasting business operations which results in optimal data value extraction.
Visvero enables businesses to reach optimal value from their analytics infrastructure. The combination of years in operation with established techniques and sustained dedication to successful outcomes lets Visvero deliver efficient and budget-friendly solutions. Their Agile Analytics Success Framework simplifies implementation while optimizing costs. Visvero delivers timely, data-driven insights that empower organizations.
Partner with Visvero and achieve analytics success today!
6. FAQs
6.1.How can businesses reduce the cost of analytics infrastructure?
Businesses can lower costs by using cloud-based solutions, automating data workflows, and adopting open-source tools. Optimizing storage and compute power is crucial for cost optimization in analytics infrastructure. Regular audits help identify unused resources, ensuring better expense management. Selecting the right pricing models for cloud services prevents overspending. Additionally, businesses should explore hybrid cloud approaches to balance cost and performance. Training staff on cost-efficient practices also helps in reducing overall infrastructure expenses.
6.2. What tools simplify analytics implementation?
Cloud-based platforms like AWS, Azure, and Google Cloud provide scalable and efficient solutions for analytics. Tools like Apache Airflow and Talend help automate data pipelines, making reducing implementation complexity in data analytics easier. Low-code and no-code platforms enable non-technical users to set up and manage analytics solutions. Managed services like Snowflake and BigQuery further simplify deployment. Selecting the right tools minimizes technical challenges, improves efficiency, and reduces overall implementation efforts.
6.3. How does cloud migration impact analytics costs?
Migrating to the cloud eliminates the need for expensive on-premise servers, reducing capital expenditures. Businesses pay for only the resources they use, ensuring cost optimization in analytics infrastructure. However, without proper monitoring, cloud costs can escalate. Organizations should adopt cost control strategies like reserved instances, autoscaling, and usage analytics. Cloud providers also offer built-in security and compliance features, reducing additional expenses. While initial migration may require investment, long-term benefits outweigh the costs.